Recently, we had the opportunity to speak with John Chapin, who is teaching CT 2.0 and developing a course around it. His work is focused on moving from traditional CT 1.0 approaches —centered on rule-based programming—to include the more dynamic, data-driven skills of CT 2.0. This shift is critical as we look to prepare students for a future where machine learning, AI, and understanding uncertainty in data play a central role.
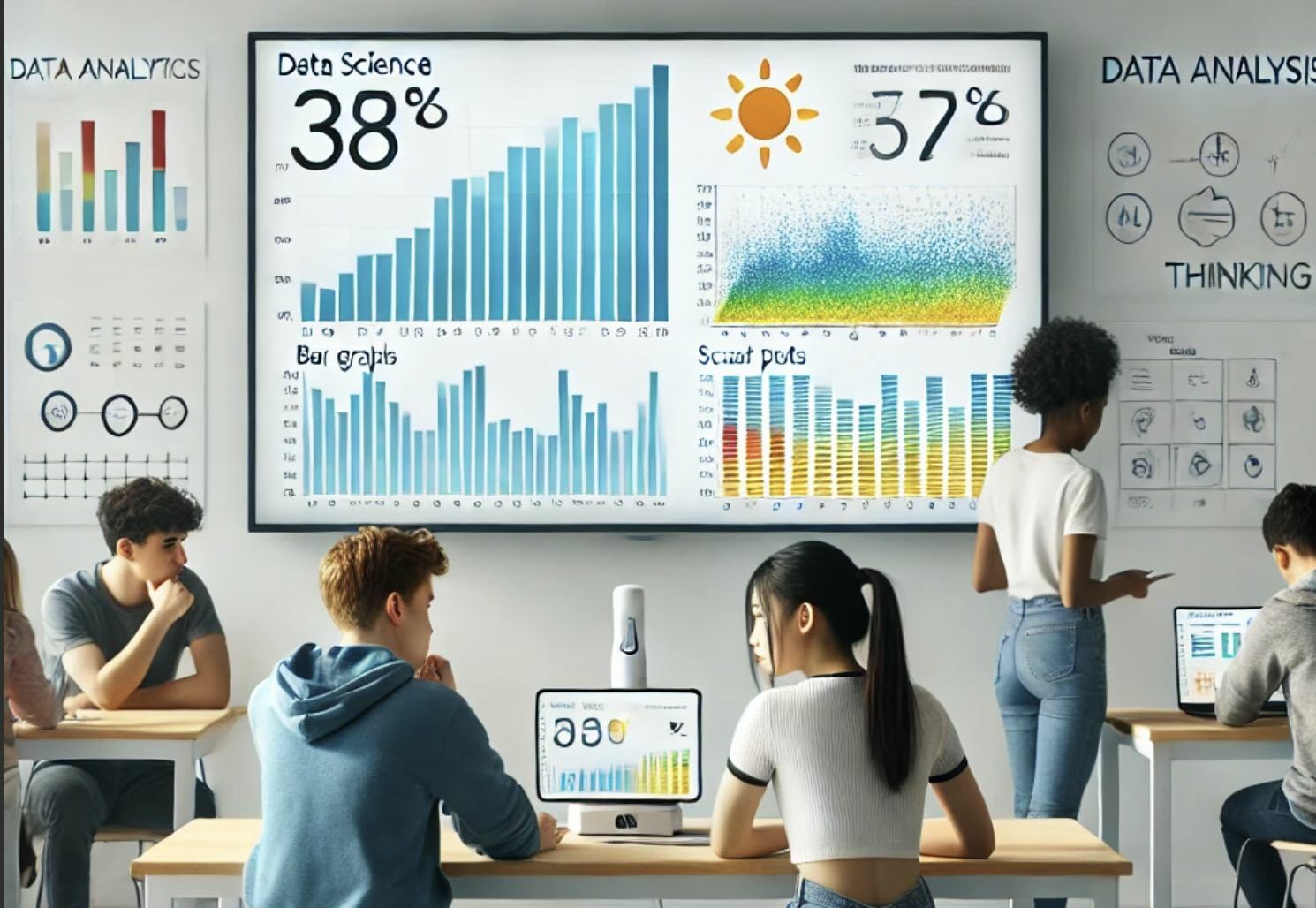
Computational Thinking 1.0 (CT 1.0) teaches programming and problem-solving through rule-based systems. Students learn to break problems down, identify patterns in repetitive tasks, and design algorithms that guide the computer through predictable sequences of operations. The focus is on algorithmic thinking and control structures like loops and conditionals, enabling students to predict the outcome of each line of code before it runs.
Computational Thinking is the foundation of computer science education, providing students with essential skills like problem decomposition, pattern recognition, and algorithm design. While these skills are crucial for algorithmic programming, the fast-paced advancements in technology mean students now need both CT 1.0 and the ability to work with data-driven, adaptive models like machine learning and AI.
The rise of data-driven solutions, artificial intelligence, and machine learning has reshaped computing, requiring educators to expand beyond traditional CT 1.0 skills. Computational Thinking 2.0 (CT 2.0) reflects this shift from rule-based programming to probabilistic, data-driven approaches that define modern applications. As [Matti Tedre and Henriikka Vartiainen]((https://www.raspberrypi.org/blog/machine-learning-education-school-computational-thinking-2-0-research-seminar/)) explain, machine learning opens new avenues for problem-solving, where students engage with uncertainty and data-driven decisions, rather than relying solely on deterministic outcomes.
An important distinction between CT 1.0 and CT 2.0 lies in their problem-solving methods: CT 1.0 relies on predetermined rules and fixed outcomes, while CT 2.0 embraces uncertainty and probability. In CT 2.0, students learn to:
- Collect and analyze large datasets
- Train models to recognize patterns
- Make predictions using statistical analysis
- Manage probabilistic outcomes
- Consider the ethical implications of AI systems
Machine learning and AI are central to CT 2.0, transforming how students approach problems. Modern computing often requires both methodologies. For example, a recommendation system may use traditional programming for its interface while relying on machine learning algorithms to generate personalized suggestions. This highlights why students need proficiency in both—understanding how to write deterministic programs and work with adaptive, data-driven models.
The ability to navigate unpredictability, especially in AI models, is essential. Mastering both rule-based programming and data-driven modeling equips students with a versatile toolkit to tackle contemporary technological challenges. By blending traditional logic with adaptive learning systems, students gain the skills to handle the complex, evolving demands of modern computing.
Here is a comparison of CT 1.0 and CT 2.0 skills. This side-by-side overview highlights the key differences between rule-based programming in CT 1.0 and data-driven, adaptive modeling in CT 2.0, offering a comprehensive framework for understanding how both approaches complement each other in contemporary computing education.
Is there a Framework that works for both?
The K-12 Computer Science Framework primarily focuses on CT 1.0 principles like algorithms, computing systems, and traditional data analysis. It provides a solid foundation for rule-based programming with predictable, step-by-step solutions.While the framework is rooted in CT 1.0, it does touch on some CT 2.0 elements, especially in its discussions on the societal and ethical impacts of computing and data handling, including networks and distributed systems.
However, the framework doesn’t fully cover advanced CT 2.0 topics, like machine learning or managing uncertainty in data models. Practices like problem identification, abstraction, and creating computational artifacts remain relevant to both CT 1.0 and CT 2.0, as students must break down problems and develop solutions, whether they’re working with algorithms or data-driven models.
Educators can use several additional frameworks and resources to guide students through essential CT2.0 skills.
- ISTE Computational Thinking Competencies: The ISTE framework is designed to integrate computational thinking across all K-12 subjects, not just computer science. While it touches on both CT 1.0 and CT 2.0 concepts, it leans more heavily towards CT 1.0, focusing on algorithmic thinking and structured problem-solving. It does include elements of CT 2.0, particularly through competencies like "Creativity and Design" and "Integrating Computational Thinking," which promote data-driven, adaptive problem-solving across various disciplines.
- Experience AI Framework: Developed with Google DeepMind, this framework introduces AI and machine learning to students aged 11-14. It focuses on CT 2.0 elements, such as shifting from rule-based to data-driven problem-solving. Students explore AI applications, machine learning models, and topics like bias and ethics in AI while learning the AI data lifecycle, which provides hands-on experience with real-world data processing.
- AI Literacy Framework: Developed by Digital Promise, helps students engage responsibly with AI systems by covering key CT 2.0 areas like data privacy, AI system evaluation, and ethical use. It offers a comprehensive approach to understanding, evaluating, and using AI systems responsibly. By addressing many ethical and practical considerations central to CT 2.0, this framework equips students to interact with AI systems in an informed and responsible manner.
- UNESCO's AI Competency Framework: While not explicitly labeled CT 2.0, this framework aligns closely with CT 2.0 principles by focusing on AI systems' critical consumption and responsible creation. It stresses AI technologies' social and ethical impacts, encouraging students to deeply engage with how AI works and its effects on society.
- Research by Matti Tedre and Henriikka Vartiainen: These researchers introduced the concept of CT 2.0 in education, shifting focus from algorithms to data-driven problem-solving. They emphasize the need to understand uncertainty in data models and develop inductive reasoning, both essential for working with AI and machine learning systems.
Educators may need to integrate multiple frameworks to fully cover both CT 1.0 and CT 2.0 skills. The K-12 Computer Science Framework offers a strong foundation for CT 1.0, while frameworks like the ISTE Computational Thinking Competencies, the Experience AI Framework, and the AI Literacy Framework provide valuable support for CT 2.0 concepts. Together, these resources help educators address the complete range of computational thinking skills, from traditional rule-based programming to modern, data-driven approaches involving AI and machine learning.
Python is Perfect for both CT 1.0 and CT 2.0
Python is ideal for teaching both CT 1.0 and CT 2.0 because it combines simplicity with the capability needed for more advanced topics. In CT 1.0, where students focus on core programming skills like algorithms, control structures, and problem decomposition, Python's clear syntax makes it easy for them to grasp foundational concepts without being overwhelmed by complex language rules. It's practical for introducing loops, conditionals, and functions, allowing students to concentrate on solving problems and building their algorithmic thinking.
As students transition to CT 2.0, Python remains a strong choice. Its wide range of tools and libraries, such as Matplotlib, NLTK, TensorFlow, scikit-learn, and Keras,, provides the resources needed to explore machine learning, AI, and data-driven models. These libraries give students hands-on experience with real-world data, enabling them to build image classifiers, create recommendation systems, and analyze large datasets. This makes Python a seamless bridge between the structured, rule-based approach of CT 1.0 and the flexible, data-driven thinking required for CT 2.0.
Beyond the classroom, Python’s widespread use in industries like healthcare, finance, and technology means students are developing valuable, real-world skills. By mastering Python in both CT 1.0 and CT 2.0, students learn how to write efficient algorithms while also working with advanced AI-driven applications, preparing them for future careers.
To further support educators, here are some resources for teaching Python:
- For CT 1.0: "Introduction to Computer Science and Programming Using Python" on edX (offered by MIT).
- For CT 1.0 and Learning Python Basics: "Python for Everybody Specialization" (Coursera)
- For CT 2.0: "Machine Learning with Python" on Coursera (offered by IBM).
- To bridge both, use "Python for Data Science and Machine Learning Bootcamp" on Udemy.
While Python is a powerful tool for both CT 1.0 and CT 2.0, it's worth noting that some educators may prefer using block-based languages like Scratch to introduce younger students to computational thinking concepts, especially when focusing on CT 1.0.
The integration of CT 1.0 and CT 2.0 is crucial for preparing students for the future. While traditional computational thinking skills remain essential, adding data-driven approaches and AI literacy rounds out the educational experience. By combining both methods and leveraging tools like Python, educators can equip students with foundational programming skills alongside the adaptive, probabilistic thinking they'll need in an AI-driven world. This approach ensures that students can solve current problems and are ready to face the new challenges brought by emerging technologies.